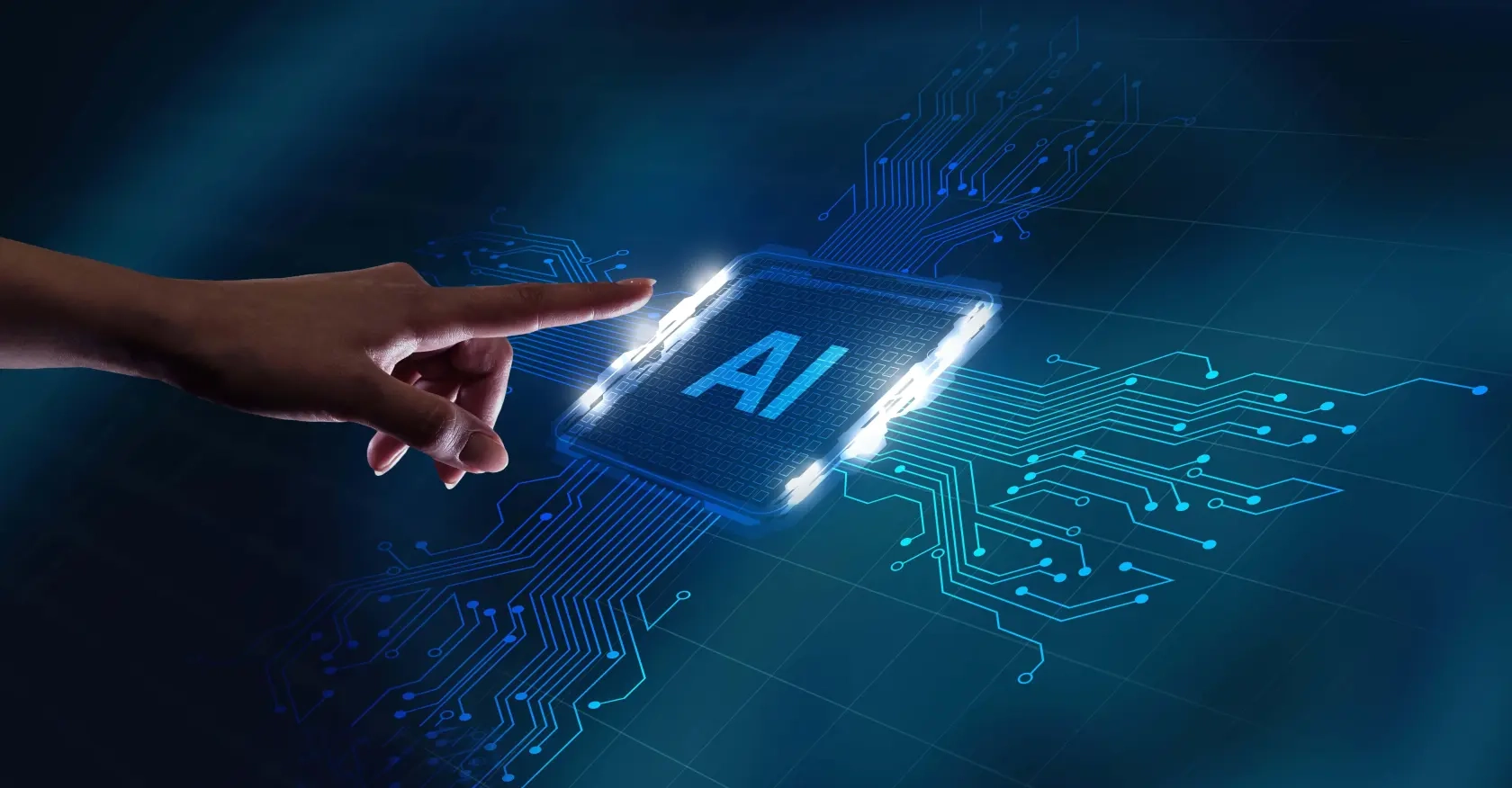
AI-Driven Energy Consulting Services
Transforming the Energy Sector with AI-Driven Solutions
The energy sector is undergoing a transformative shift, driven by global demands for efficiency and sustainability. As this industry navigates its evolving challenges, the application of Artificial Intelligence (AI) is emerging as an invaluable tool. Meticulous, data-driven, and ever-advancing, AI consultancy services provide evidence-based solutions that prioritize scientific accuracy, optimizing various facets of energy production, distribution, and consumption.
AI-driven Consultancy Services for the Energy Sector
AI-driven consultancy services tailored specifically for the energy sector, detailing the techniques, algorithms, infrastructure, statistical models, and best-fit scenarios:
AI-Enhanced Energy Demand Forecasting
- Techniques: Deep Learning with Recurrent Neural Networks (RNN) and Long Short-Term Memory (LSTM) models for sequential data.
- Algorithms: Time series analysis and forecasting algorithms to predict energy consumption patterns.
- Infrastructure: Smart meters, cloud-based platforms for real-time data processing and storage.
- Statistical Models: Regression models, seasonal decomposition.
- Fit: Utility providers, grid operators, and energy market traders.
Carbon Footprint Analysis & Reduction
- Techniques: Regression models and Decision Trees.
- Algorithms: Analysis of energy consumption patterns to calculate carbon footprint; optimization algorithms for suggesting reduction methods.
- Infrastructure: Sensors measuring emissions, databases of global emission factors, cloud servers for analytics.
- Statistical Models: Regression for footprint prediction, classification for identifying high-emission activities.
- Fit: Industries aiming for sustainability, governmental bodies, environmental agencies.
Renewable Energy Optimization
- Techniques: Reinforcement Learning for dynamic system optimization.
- Algorithms: Predictive models to determine optimal energy storage and release times based on demand and weather forecasts.
- Infrastructure: IoT sensors on solar panels and wind turbines, battery storage data analytics systems.
- Statistical Models: Regression analysis, trend prediction.
- Fit: Renewable energy producers, especially in solar and wind sectors.
Smart Grid Management
- Techniques: Neural Networks and Decision Trees for decision-making processes.
- Algorithms: Optimization algorithms for efficient energy distribution and storage.
- Infrastructure: Smart grid hardware, real-time data transmission systems, centralized control centers.
- Statistical Models: Classification models for fault detection, clustering for consumer grouping.
- Fit: National and regional grid operators, municipal electricity boards.
Predictive Maintenance for Energy Infrastructure
- Techniques: Convolutional Neural Networks (CNN) for image processing; Natural Language Processing (NLP) for analyzing maintenance logs.
- Algorithms: Anomaly detection to predict equipment failures.
- Infrastructure: IoT sensors on energy infrastructure, cameras for visual inspections, cloud servers for data analytics.
- Statistical Models: Time series anomaly detection, classification models for fault identification.
- Fit: Power plants, grid operators, and oil & gas companies.
AI in Energy: A Quick Dive
Artificial Intelligence (AI) is reshaping the energy sector, offering solutions for efficiency, sustainability, and adaptability. Here’s a swift look into AI-driven energy services:
- Demand Forecasting: Uses RNN and LSTM models. Key Infrastructure: Smart meters.
- Renewable Energy: Optimization via Reinforcement Learning. Infrastructure highlight: IoT sensors on solar panels.
- Smart Grids: Managed with Neural Networks. Critical infrastructure: Smart grid hardware.
- Maintenance: Predictive with CNN and NLP. Main Infrastructure: IoT sensors, cameras.
- Battery Optimization: Deep Learning for battery interactions. Key tool: Embedded sensors.
- Market Forecasting: Uses SVM and ARIMA. Infrastructure highlight: Real-time market data feeds.
- Carbon Reduction: Regression models for analysis. Key tool: Emission sensors.
Each service merges energy expertise with AI’s precision, addressing diverse energy challenges from plant level to national grids.
To encapsulate, the trajectory of the energy sector is intrinsically linked with its capacity for technological integration. The implementation of AI-driven consultancy services, characterized by rigorous scientific techniques and objective methodologies, is proving crucial in addressing contemporary energy challenges. By leveraging the precise analytical capabilities of AI, the industry is better positioned to make informed decisions, ensuring both operational efficiency and adherence to sustainable practices.
For Ecolonical TECH Stakeholders: Recognizing the intersection of ecological concerns and technological solutions is paramount in today’s energy landscape. If you’re seeking to explore the potential benefits of AI in the energy sector, we’re here to assist. Let’s collaborate to understand and harness these capabilities for your specific needs, contact us to learn more.